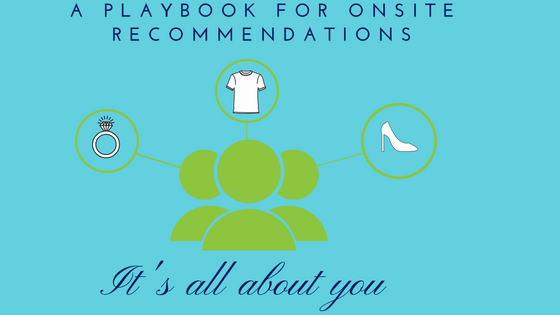
The role of recommendations in an e-commerce store is very similar to that of the salesperson in a brick and mortar store. Let’s draw a comparison between both experiences.
In your visit to a boutique, you are greeted by a salesman who chats you up to obtain a notion of what interests you and guides you to that section of products. He displays the most eye-catching pieces in that section. This is your initial encounter with the products. In an online experience, this interaction is captured by high-level recommendations like Trending products, Best Sellers and New Arrivals at both site-level and category-level.
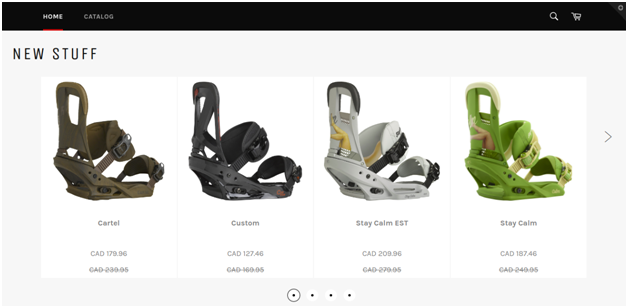
Continuing with our boutique experience, after browsing the products you would express interest in a few products that catch your eye. A salesman reads this and starts bringing you products similar to the products that you are interested in. From this point on your interaction with the products that interest you maximizes, so the chances of you making a purchase are high. This interaction is captured by product-specific recommendations like similar products to this product, people who were interested in this product were also interested in these.
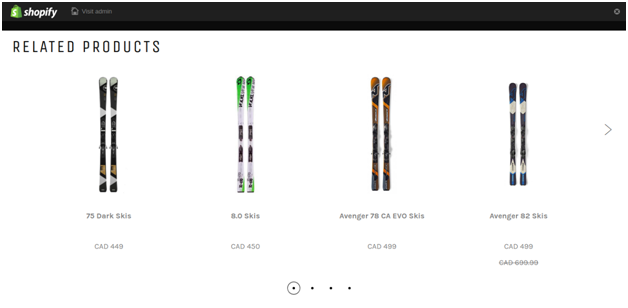
After you’ve finalized the products that you are purchasing, the salesman would now show you products that exactly pair up with the products you are purchasing, and since the products go well with your soon to own products, you could probably be coaxed into buying few those products too. This last-minute temptation is taken care of by cart recommendations which are similar products to the products in your cart shown at checkout.
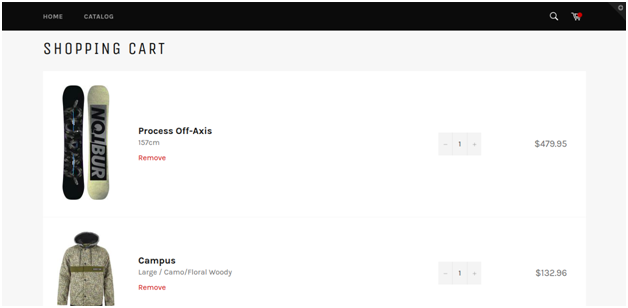
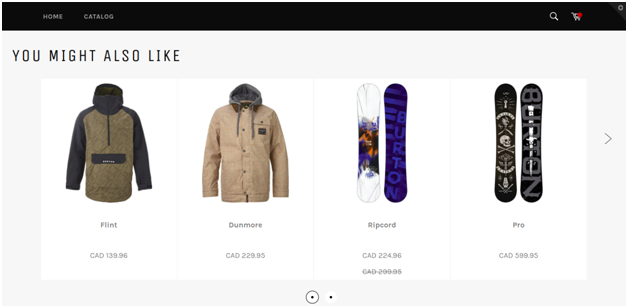
From this comparison, we can see that we’ve captured most of the interactions that happen during our first visit to a brick and mortar store. Now let’s look at a second visit to the same store. Now if you weren’t particularly high-paying or a highly interested person the chances of the salesman remembering you are slim. But let’s say that he does remember you, then your shopping experience gets better by magnitudes, from the starting point you are shown the products that match your taste. This is captured by user-level personalized recommendations, and as a bonus, it is not biased towards the interesting ones. Imagine the immense effect it will have on you as a customer if every brick and mortar store you revisit is rearranged to exactly match your taste, this is what personalized recommendations do to your e-commerce store.
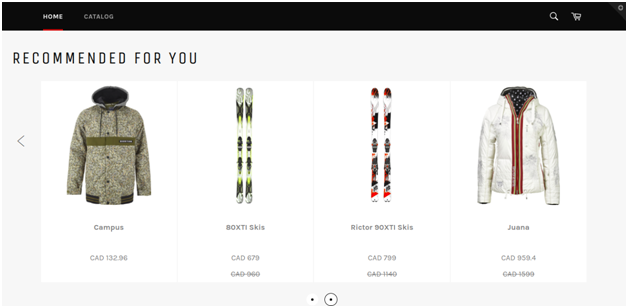
Getting the recommendations strategy right
If you’ve interacted with e-commerce stores other than the Amazons and Flipkarts of the world, you would rarely come across a store that checks the box on all types of recommendations mentioned above. If every e-commerce store realizes the boost provided by on-site recommendations, what’s stopping them from using them? The answer most often is the lack of data. To workaround this data problem, and strategize on what recommendation to use based on what part of the e-commerce journey your store is in, these recommendation playbooks will come in handy.
Playbook 1 – I’ve launched my e-commerce store, what next?
At this stage, getting the customer journey through your site right is of utmost importance, and the recommendation widgets help in this regard by increasing the navigability of the site. Having recommendations puts more products in front of the user. One important metric to track the success of recommendations at this stage is the average number of product interactions per customer. With the customer activity being low on the site, at this stage the data at hand – catalog data – acts as the basis for recommendations. When you do not have enough customer activity on your site but have a rich catalog of products, focus on showcasing products and improving their discoverability. Use product similarity algorithms to offer more choices.
High-level recommendations – They can be used to exhibit individual products on your site’s landing page and category landing pages. A good thing about these recommendations is that use-cases like new arrivals, trending products, and best-sellers work by capturing the overall trend in the store and provides good recommendations with fewer data.
Product-specific recommendations – The recommendation based on users like people who bought this also viewed, might not converge well to provide good recommendations for all products. But having recommendations on product pages is important to increase the site’s navigability. At this stage to have product-specific recommendations, we could leverage the catalog data to provide content-based recommended products, without using user-based data. These recommendations are learned from the product data like name, description, and other attributes present in the catalog. This can be very powerful in the initial stages of the e-commerce store. Going by our salesman analogy, the salesman learns by comparing products before learning about the customers’ taste. The same content-based similarity approach can be used for populating cart recommendations
Personalized recommendations – Similar to product-specific recommendations we could leverage the content, to generate personalized recommendations. For example, ‘Spiderman-superhero-t-shirt‘ can be recommended to a user, who has bought ‘Ironman-superhero-t-shirt‘. Because from the IDs we can gauge a high similarity score between the products
Playbook 2 – Traffic is picking up, Orders are placed, efforts are taken to optimize the conversion funnel.
With the right recommendations in the previous stage, we would have gathered data on valuable customer behavior patterns in the store. Huge improvements can be made to the recommendations by leveraging this data.
High-level recommendations – The general quality of the recommendations like best-sellers and trending products will have improved with the volume of data we have now. At this point, we could filter the general widgets to have only the use-cases that work best. Their positions on the website can be re-assigned to more relevant recommendations like personalized recommendations.
Product-specific recommendations – We could now start using user activity-based recommendations like people who viewed this also viewed, bought this also bought. While the content-based recommendations capture the similarity between products by content, these recommendations capture similarity based on user intent and are capable of uncovering more not so apparent relationships between products in the catalog. This can also help in promoting cross-sell between categories and across verticals.
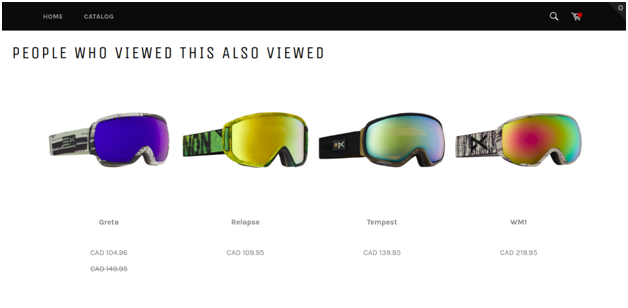
Personalized recommendations – A good user-activity based personalization algorithm usually requires a good amount of training data to perform competently against content-based personalization algorithms. At this stage, this will depend on metrics such as average order basket size, the average number of product interactions per customer, etc. Therefore, instead of a complete switch to user-activity based personalization, a mix of both content-based and user-activity based personalization can be used.
Playbook 3 – Achieved scale, emerged as a significant player. Trying to carve up a bigger portion of the market share pie.
Your site has now reached a scale to an ecosystem of its own, one which is unique in every way, with its own set of unique opportunities waiting to be uncovered and a unique set of problems to be solved. At this stage it’s high time to analyze whether every ounce of useful data is being extracted from the ecosystem, implicit signals like, time spent on each listing, sharing of each listing, reviews, wishlists, points of exit, etc. can be used to make the recommendations better.
High-level recommendations – By now personalized recommendations can take the mainstay in the site’s landing page and category landing page. The general use cases can be used as a fallback to personalized recommendations to handle new users. And improvements to the recommendations by using implicit data – location, time of the day, etc – can be explored.
Product-specific recommendations – At this stage, we have enough data to significantly rely on user activity to provide product-specific recommendations. To further optimize the performance of the recommendations, we could experiment with sampling techniques based on product quality, etc.
The content-based similarity will still have its place while new products are added to the catalog. Content-based similarity can also be made better by taking into account the image similarity between products. Look out for significant affinity between products across categories to uncover cross-sell opportunities for cart recommendations. The trends learned from these activities can also influence your discounting strategy.
Personalized recommendations – Personalization can be the potential differentiator and provide an edge over your competitors at this stage. It’s worth exploring if a bespoke personalization algorithm that excels at capturing trends specific to the industry, like Fashion, electronics, jewelry, etc., would be a good replacement for the common algorithms based on collaborative filtering. And the act of personalization no longer needs to be limited to widgets, it can be interwoven into every activity the user undertakes. Search personalization, Deals personalization, etc. can also be undertaken.
With these strategies in place, recommendations can provide a huge boost to your site. These strategies also help in settings the expectations around recommendations based on the stage you are in. One issue that can be particularly tricky in this journey, is to decide when to make the shift from one strategy to another without affecting the relevance of the current recommendations. We at YFret provide a unified solution in our platform for the first two strategies and could work with you if you are looking to solve specific data-related problems on your website.
#personalizedrecommendations #websitepersonalization #websiterecommendations